第一代mapred调度架构
mapredv1 scheduler 分析
第一代 mapred 调度器是通过实现 TaskScheduler
接口来提供的,是一个可插拔的接口,用户可以定义自己的调度器,并通过配置文件指定。通过反射机制,可以加载调度器。但是,由于第一代 mapred 计算框架的设计的原因,调度方式其实受到 JobTracker
中资源表示和管理的严格限制。
调度的资源表示模型采用的是 基于静态的固定分类的slots表示方法,将多维度的 CPU/MEM 等资源降维成一个维度的 slot,slot 中 CPU和MEM 大小则是通过配置得到。而每种 Task 需要的资源都是通过 slots 来表示。TaskTracker 上拥有 map slots 和 reduce slots, Task 需要多少 slots 由程序指定。这也意味着, 每个节点上的slot数目决定了该节点上的最大允许的任务并发度。 为了区分Map Task和Reduce Task所用资源量的差异, slot又被分为Map slot和Reduce slot两种, 它们分别只能被Map Task和Reduce Task使用。 Hadoop集群管理员可根据各个节点硬件配置和应用特点为它们分配不同的Map slot数(由参数mapred.tasktracker.map.tasks.maximum指定) 和Reduce slot数(由参数mapred.tasktracker.reduce.tasks.maximum指定) 。
比如, 管理员事先规划好一个slot代表2 GB内存和1个CPU, 如果一个应用程序的任务只需要1 GB内存, 则会产生“资源碎片”, 从而降低集群资源的利用率; 同样, 如果一个应用程序的任务需要3 GB内存, 则会隐式地抢占其他任务的资源, 从而产生资源抢占现象, 可能导致集群利用率过高。 因此, 寻求一种更精细的资源划分方法显得尤为必要。
在Hadoop中, 由于Map Task和Reduce Task运行时使用了不同种类的资源(不同种类的slot) , 且这两种资源之间不能混用, 因此任务调度器分别对Map Task和Reduce Task单独进行调度。 而对于同一个作业而言, Reduce Task和Map Task之间存在数据依赖关系, 默认情况下, 当Map Task完成数目达到总数的5%(可通过参数mapred.reduce.slowstart.completed.maps配置) 后, 才开始启动Reduce Task(ReduceTask开始被调度).
第一代 mapred 提供了一个默认的调度器,org.apache.hadoop.mapred.JobQueueTaskScheduler
,也是一个FIFO作业调度器,实现非常简单。
调度器调度的时候,并不是主动行为,而是每一个节点 TaskTracker
通过心跳的方式从 JobTracker
拿到任务列表,而 JobTracker
则利用调度器的 assignTasks
方法进行调度。也就是说调度器每次只处理一个 TaskTracker
的任务调度请求,他负责遍历队列中的作业,寻找作业中合适的任务分配给 TaskTracker
,下图是作业提交到执行的过程。
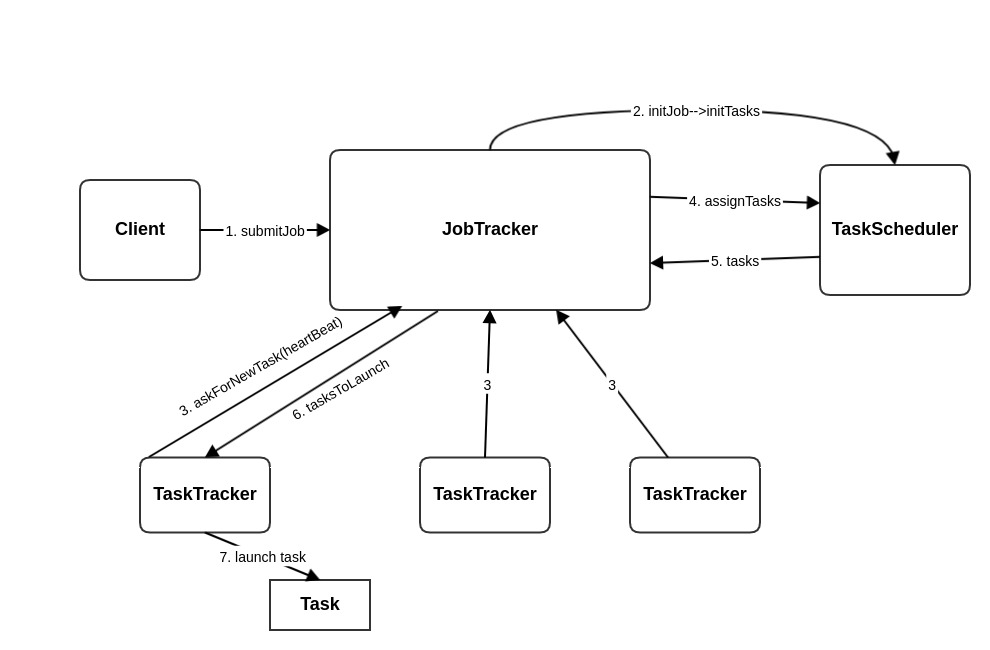
是节点向 JobTracker
申请 Task
,而不是任务被提交后就可以立即由调度器进行调度分配,Hadoop 采用这种模式,我认为主要是因为第一,离线任务都属于耗时任务,任务提交后排队延迟和调度时间相对于任务本身执行时间忽略不计, 而像公有云虚拟机调度,是希望调度能够立即响应出分配策略的,因此采用了主控制器主动分配给某个Host节点,而不是由Host节点主动通过心跳包拉取可分配的任务(创建虚拟机,相对来说创建虚拟机是比较快的,基本在几分钟左右,容器则更快,几十秒即可); 第二, JobTracker
和 TaskTracker
之间是通过 RPC 协议通讯的,这里 JobTracker
对于 TaskTracker
来说,扮演的其实是服务器的角色。JobTracker
不会去主动调用 TaskTracker
。
TaskScheduler
和 JobTracker
之间其实是通过观察者模式来实现的,如图 JobTracker-TaskScheduler-观察者模式 所示,JobTracker
作为被观察者,其实真正的观察者是 JobInProgressListener
, TaskScheduler
只是利用了注册在 JobTracker
中的 JobInProgressListener
来获取作业队列。在默认调度器中,队列其实是由JobQueueJobInProgressListener.jobQueue
数据结构来维护,他其实是 TreeMap
,key 是 JobSchedulingInfo
, 通过 开始时间 排序。
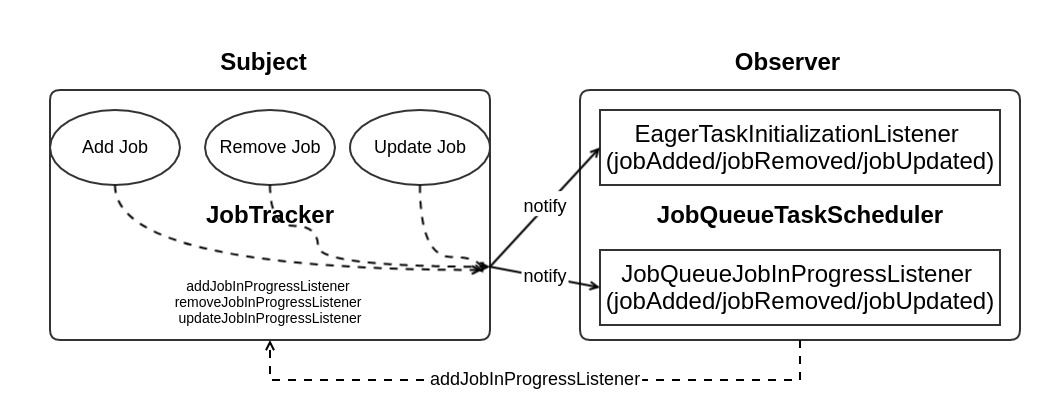
Job 的添加/删除 JobTracker
都会第一时间通知观察者,addJob
就是通知观察者有新 Job 加入的函数,观察者会触发相应的动作。
private synchronized JobStatus addJob(JobID jobId, JobInProgress job) throws IOException { totalSubmissions++; synchronized (jobs) { synchronized (taskScheduler) { jobs.put(job.getProfile().getJobID(), job); for (JobInProgressListener listener : jobInProgressListeners) { listener.jobAdded(job); } } } myInstrumentation.submitJob(job.getJobConf(), jobId); job.getQueueMetrics().submitJob(job.getJobConf(), jobId); ...... return job.getStatus(); }
比如 JobTracker
在添加一个 Job的时候,对于默认调度器而言,调度器中的 EagerTaskInitializationListener
是通过线程池完成对作业的初始化的。
Task
, TaskTracker
, Node
的物理视图。 一个 Node
可以含有多个 TaskTracker
, 但是通常而言是一个; 一个 TaskTracker
控制多个 Task
, 而 Task
运行所在的 Node
一定是 TaskTracker
所在的Node
吗?
答案是否定的,Task
不一定运行在管理它的 TaskTracker
所在节点运行,例如有些 Task
不需要考虑 data locality, 可能它的 InputSplitLocation
是空的; 下图是 Task
, TaskTracker
, Node
的物理运行视图。
一个Task可使用多少slot完全由调度器决定。 当前大部分调度器只支持一个Task占用一个slot, 比如FIFO和Fair Scheduler; 而Capacity Scheduler则可根据Task内存需求为其分配多个slot。
JobQueueTaskScheduler
调度过程如下:
1. 获取整个集群的容量以及当前请求的 TaskTracker 容量。
2. 计算目前整个集群能达到的最大负载峰值。通过计算出所有在运行以及将要运行的任务,即总需求量,除以整个集群的容量即可求出负载因子。
int remainingReduceLoad = 0; int remainingMapLoad = 0; synchronized (jobQueue) { for (JobInProgress job : jobQueue) { if (job.getStatus().getRunState() == JobStatus.RUNNING) { remainingMapLoad += (job.desiredMaps() - job.finishedMaps()); if (job.scheduleReduces()) { remainingReduceLoad += (job.desiredReduces() - job.finishedReduces()); } } } } // Compute the 'load factor' for maps and reduces double mapLoadFactor = 0.0; if (clusterMapCapacity > 0) { mapLoadFactor = (double)remainingMapLoad / clusterMapCapacity; } double reduceLoadFactor = 0.0; if (clusterReduceCapacity > 0) { reduceLoadFactor = (double)remainingReduceLoad / clusterReduceCapacity; }
- 计算当前 TaskTracker 可分配的空闲资源,这里采用的策略是不能超过负载峰值,即 TaskTracker 的空闲资源是其容量乘于负载因子,而不是真实的容量。这也是为了保证集群的负载均衡,让所有TaskTracker 尽可能保持均衡的资源消耗。
final int trackerCurrentMapCapacity = Math.min((int)Math.ceil(mapLoadFactor * trackerMapCapacity), trackerMapCapacity); int availableMapSlots = trackerCurrentMapCapacity - trackerRunningMaps;
- 遍历队列中的作业,尝试将所有可分配的任务分配给该 TaskTracker. v1 中的任务选择策略是一样的, hadoop 将之封装在通用模块中供各种调度器使用,具体就是调用
JobInProgress.obtainNewNodeOrRackLocalMapTask
或者JobInProgress.obtainNewNonLocalMapTask
来获取可分配任务。这些函数最终调用的是findNewMapTask
, 通过采用网络拓扑和数据本地性策略来分配。
@Override public synchronized List<Task> assignTasks(TaskTracker taskTracker) throws IOException { // 这里整个集群数据 ClusterStatus 的获取其实并不是实时的,上一个TaskTracker调用 `assignTasks` 以后, /// 可能 TaskTracker 的资源减少了,但是 ClusterStatus还未及时更新数据 TaskTrackerStatus taskTrackerStatus = taskTracker.getStatus(); ClusterStatus clusterStatus = taskTrackerManager.getClusterStatus(); final int numTaskTrackers = clusterStatus.getTaskTrackers(); final int clusterMapCapacity = clusterStatus.getMaxMapTasks(); final int clusterReduceCapacity = clusterStatus.getMaxReduceTasks(); Collection<JobInProgress> jobQueue = jobQueueJobInProgressListener.getJobQueue(); // // Get map + reduce counts for the current tracker. // final int trackerMapCapacity = taskTrackerStatus.getMaxMapSlots(); final int trackerReduceCapacity = taskTrackerStatus.getMaxReduceSlots(); final int trackerRunningMaps = taskTrackerStatus.countMapTasks(); final int trackerRunningReduces = taskTrackerStatus.countReduceTasks(); // Assigned tasks List<Task> assignedTasks = new ArrayList<Task>(); // // Compute (running + pending) map and reduce task numbers across pool // int remainingReduceLoad = 0; int remainingMapLoad = 0; synchronized (jobQueue) { // jobQueue 枷锁,防止读取和写入的并发操作 for (JobInProgress job : jobQueue) { if (job.getStatus().getRunState() == JobStatus.RUNNING) { remainingMapLoad += (job.desiredMaps() - job.finishedMaps()); if (job.scheduleReduces()) { remainingReduceLoad += (job.desiredReduces() - job.finishedReduces()); } } } } // Compute the 'load factor' for maps and reduces double mapLoadFactor = 0.0; if (clusterMapCapacity > 0) { mapLoadFactor = (double)remainingMapLoad / clusterMapCapacity; } double reduceLoadFactor = 0.0; if (clusterReduceCapacity > 0) { reduceLoadFactor = (double)remainingReduceLoad / clusterReduceCapacity; } // // In the below steps, we allocate first map tasks (if appropriate), // and then reduce tasks if appropriate. We go through all jobs // in order of job arrival; jobs only get serviced if their // predecessors are serviced, too. // // // We assign tasks to the current taskTracker if the given machine // has a workload that's less than the maximum load of that kind of // task. // However, if the cluster is close to getting loaded i.e. we don't // have enough _padding_ for speculative executions etc., we only // schedule the "highest priority" task i.e. the task from the job // with the highest priority. // final int trackerCurrentMapCapacity = Math.min((int)Math.ceil(mapLoadFactor * trackerMapCapacity), trackerMapCapacity); int availableMapSlots = trackerCurrentMapCapacity - trackerRunningMaps; boolean exceededMapPadding = false; if (availableMapSlots > 0) { exceededMapPadding = exceededPadding(true, clusterStatus, trackerMapCapacity); } int numLocalMaps = 0; int numNonLocalMaps = 0; scheduleMaps: for (int i=0; i < availableMapSlots; ++i) { synchronized (jobQueue) { for (JobInProgress job : jobQueue) { if (job.getStatus().getRunState() != JobStatus.RUNNING) { continue; } Task t = null; // Try to schedule a node-local or rack-local Map task t = job.obtainNewNodeOrRackLocalMapTask(taskTrackerStatus, numTaskTrackers, taskTrackerManager.getNumberOfUniqueHosts()); if (t != null) { assignedTasks.add(t); ++numLocalMaps; // Don't assign map tasks to the hilt! // Leave some free slots in the cluster for future task-failures, // speculative tasks etc. beyond the highest priority job if (exceededMapPadding) { break scheduleMaps; } // Try all jobs again for the next Map task break; } // Try to schedule a node-local or rack-local Map task t = job.obtainNewNonLocalMapTask(taskTrackerStatus, numTaskTrackers, taskTrackerManager.getNumberOfUniqueHosts()); if (t != null) { assignedTasks.add(t); ++numNonLocalMaps; // We assign at most 1 off-switch or speculative task // This is to prevent TaskTrackers from stealing local-tasks // from other TaskTrackers. break scheduleMaps; } } } } int assignedMaps = assignedTasks.size(); // // Same thing, but for reduce tasks // However we _never_ assign more than 1 reduce task per heartbeat // final int trackerCurrentReduceCapacity = Math.min((int)Math.ceil(reduceLoadFactor * trackerReduceCapacity), trackerReduceCapacity); final int availableReduceSlots = Math.min((trackerCurrentReduceCapacity - trackerRunningReduces), 1); boolean exceededReducePadding = false; if (availableReduceSlots > 0) { exceededReducePadding = exceededPadding(false, clusterStatus, trackerReduceCapacity); synchronized (jobQueue) { for (JobInProgress job : jobQueue) { if (job.getStatus().getRunState() != JobStatus.RUNNING || job.numReduceTasks == 0) { continue; } Task t = job.obtainNewReduceTask(taskTrackerStatus, numTaskTrackers, taskTrackerManager.getNumberOfUniqueHosts() ); if (t != null) { assignedTasks.add(t); break; } // Don't assign reduce tasks to the hilt! // Leave some free slots in the cluster for future task-failures, // speculative tasks etc. beyond the highest priority job if (exceededReducePadding) { break; } } } } if (LOG.isDebugEnabled()) { LOG.debug("Task assignments for " + taskTrackerStatus.getTrackerName() + " --> " + "[" + mapLoadFactor + ", " + trackerMapCapacity + ", " + trackerCurrentMapCapacity + ", " + trackerRunningMaps + "] -> [" + (trackerCurrentMapCapacity - trackerRunningMaps) + ", " + assignedMaps + " (" + numLocalMaps + ", " + numNonLocalMaps + ")] [" + reduceLoadFactor + ", " + trackerReduceCapacity + ", " + trackerCurrentReduceCapacity + "," + trackerRunningReduces + "] -> [" + (trackerCurrentReduceCapacity - trackerRunningReduces) + ", " + (assignedTasks.size()-assignedMaps) + "]"); } return assignedTasks; }
任务选择策略分析
选择任务能够是否能够分配给TaskTracker,首先得把Job拆分成Tasks,然后根据相应的策略来分析是否将Task 分配给TaskTracker。Hadoop 不仅使用计算资源slot模型来调度和分配任务,而且引入网络拓扑,考虑数据本地性,网络带宽等拓扑数据进行调度。
数据本地性
Hadoop根据输入数据与实际分配的计算资源之间的距离将任务分成三类: node-local(输入数据与计算资源同节点), rack-local(同机架)和 off-switch(跨机架). 当输入数据与计算资源位于不同节点上时, Hadoop需将输入数据远程复制到计算资源所在节点进行处理。 两者距离越远,需要的网络开销越大, 因此调度器进行任务分配时尽量选择离输入数据近的节点资源。
当Hadoop进行任务选择时, 采用了自下向上查找的策略。 由于当前采用了两层网络拓扑结构, 因此这种选择机制决定了任务优先级从高到低依次为: node-local、 rack-local和off-switch。 下面结合图介绍三种类型的任务被选中的场景。
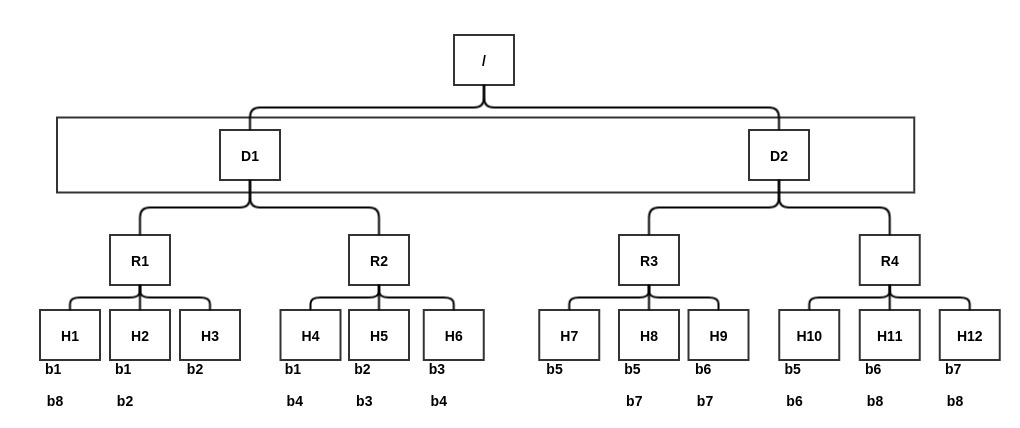
假设某一时刻, TaskTracker X出现空闲的计算资源, 向JobTracker汇报心跳请求新的任务, 调度器根据一定的调度策略为之选择了任务Y。
场景1 如果X是H1, 任务Y输入数据块为b1, 则该任务为node-local。
场景2 如果X是H1, 任务Y输入数据块为b2, 则该任务为rack-local。
场景3 如果X是H1, 任务Y输入数据块为b4, 则该任务为off-switch。
Job 和 Tasks 初始化
Job
的提交/删除/更新都是通过观察者模式来实现,客户端调用 submitJob
RPC 以后,JobTracker
就会通知所有的监听器 Job
被提交。Job
被提交之后的行为基本上通过外部调度器来完成。当然 JobRecoveryManager
也会进行Job
初始化工作。
例如 JobQueueTaskScheduler
是通过 EagerTaskInitializationListener
监听器实现初始化,并且该监听器内部维护一个 jobInitManagerThread
线程完成 Job
的初始化工作, 该线程又是通过线程池来执行要初始化的 Job
。
而 FairScheduler
则通过 JobInitializer
初始化。
JobTracker
提供了 initJob()
方法来对 Job 进行初始化,该方法调用的是 JobInProgress.initTasks()
方法。主要是设置 Job 的相关 Tasks 以及他们的具有本地数据的节点缓存。
- 设置 Job 优先级
- 生成 security key
- 根据输入文件进行分片计算,
createSplits(jobId)
创建出 Map 的分片元信息,设置 Map 任务数 numMapTasks, 这里必须和splits分片数一样 - 设置 QueueMetrics 计量
- 新建 maps TaskInProgress 任务数组
createCache()
创建nonRunningMapCache
节点本地任务缓存- 新建 reduces TaskInProgress 任务数组
- 设置 cleanup 和 setup 任务
org.apache.hadoop.mapred.JobInProgress
这里的步骤6 是利用数据本地性策略的关键,首先创建该 Map<Node, List<TaskInProgress>>
的缓存map对象,该对象中包含 每个节点可以运行的任务列表。缓存利用了网络拓扑结构,Hadoop 利用 org.apache.hadoop.net.NetworkTopology
将整个集群抽象成树形网络拓扑结构,其中 host, rack/switch/router, datacenter 都是抽象成节点 Node. Hadoop 只能感知到交换机这一层。如图 是构建该缓存的过程,maxLevel
控制着缓存的级别,第2层就是交换机这一层,第0层即使node-local, 第1层就是rack-local, 如果允许 off-switch, 则再利用缓存查询的时候,可以直接遍历到该层来获取Tasks。
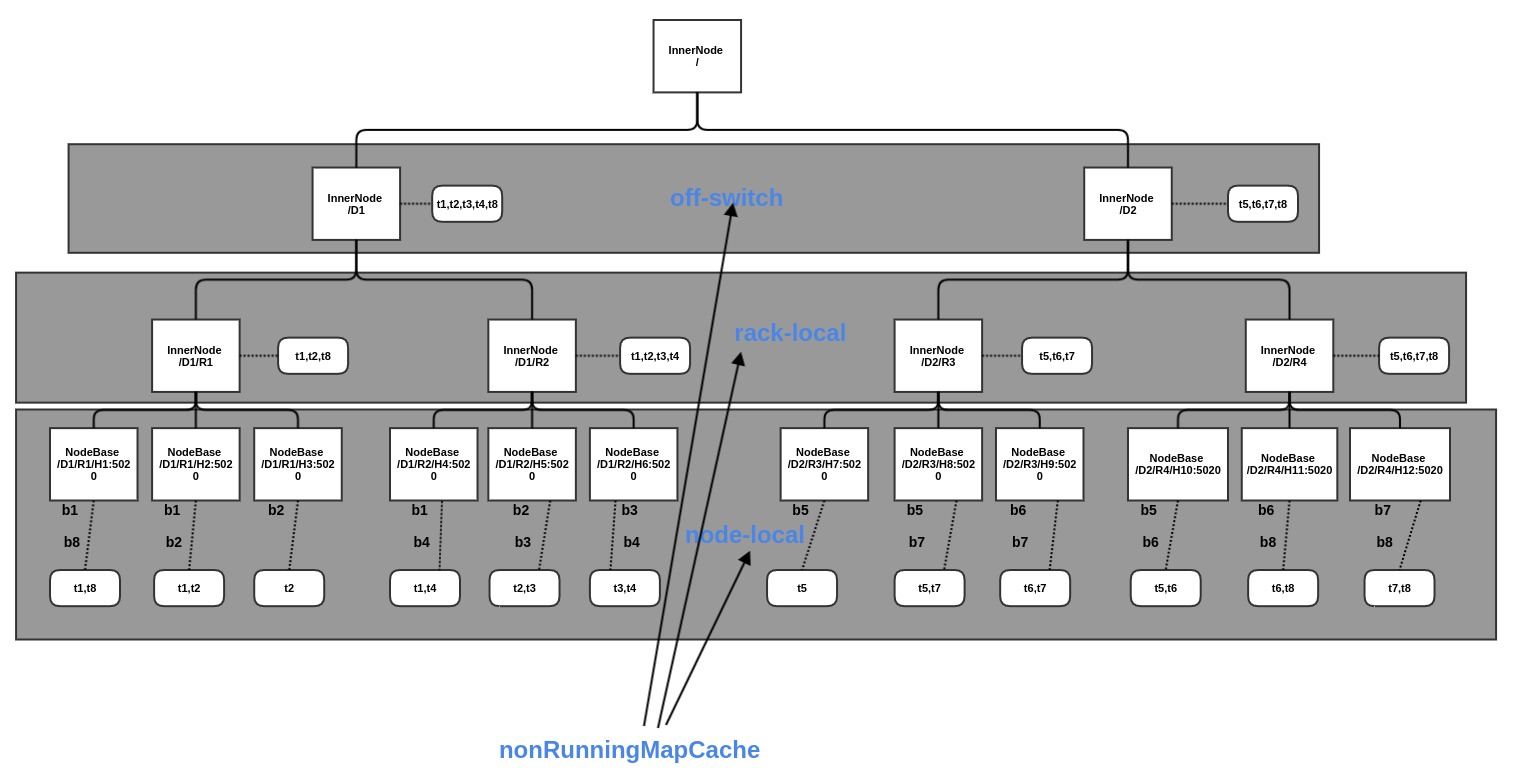
private Map<Node, List<TaskInProgress>> createCache( TaskSplitMetaInfo[] splits, int maxLevel) throws UnknownHostException { // 创建缓存对象 Map<Node, List<TaskInProgress>> cache = new IdentityHashMap<Node, List<TaskInProgress>>(maxLevel); // 每一个数据分片会对应一个MapTask,而数据分片在HDFS上都含有副本,因此可以得到某个分片splits[i]的所有副本所在的机器 splitLocations Set<String> uniqueHosts = new TreeSet<String>(); for (int i = 0; i < splits.length; i++) { String[] splitLocations = splits[i].getLocations(); if (splitLocations == null || splitLocations.length == 0) { nonLocalMaps.add(maps[i]); continue; } // 对所有含有副本数据的host,都加入到 map<node,tips> cache 缓存之中 for(String host: splitLocations) { Node node = jobtracker.resolveAndAddToTopology(host); uniqueHosts.add(host); LOG.info("tip:" + maps[i].getTIPId() + " has split on node:" + node); // 这里是对树形网络拓扑结构,将副本数据所在的顶层rack/switch/router Node 也加入缓存,这样就知道每个Node缓存适合运行的tips列表了 for (int j = 0; j < maxLevel; j++) { List<TaskInProgress> hostMaps = cache.get(node); if (hostMaps == null) { hostMaps = new ArrayList<TaskInProgress>(); cache.put(node, hostMaps); hostMaps.add(maps[i]); } //check whether the hostMaps already contains an entry for a TIP //This will be true for nodes that are racks and multiple nodes in //the rack contain the input for a tip. Note that if it already //exists in the hostMaps, it must be the last element there since //we process one TIP at a time sequentially in the split-size order // 多个数据副本可能在同一个rack之内,因此可能已经含有该rack Node 的hostMaps 了,检查是否已经加入过该maptask[i], 没有则加入 if (hostMaps.get(hostMaps.size() - 1) != maps[i]) { hostMaps.add(maps[i]); } node = node.getParent(); } } } //设置需要使用 data locality 任务的等待时间因子 // Calibrate the localityWaitFactor - Do not override user intent! if (localityWaitFactor == DEFAULT_LOCALITY_WAIT_FACTOR) { int jobNodes = uniqueHosts.size(); int clusterNodes = jobtracker.getNumberOfUniqueHosts(); if (clusterNodes > 0) { localityWaitFactor = Math.min((float)jobNodes/clusterNodes, localityWaitFactor); } LOG.info(jobId + " LOCALITY_WAIT_FACTOR=" + localityWaitFactor); } return cache; } public Node resolveAndAddToTopology(String name) throws UnknownHostException { List <String> tmpList = new ArrayList<String>(1); tmpList.add(name); List <String> rNameList = dnsToSwitchMapping.resolve(tmpList); String rName = rNameList.get(0); String networkLoc = NodeBase.normalize(rName); return addHostToNodeMapping(name, networkLoc); } // clusterMap = new NetworkTopology(); private Node addHostToNodeMapping(String host, String networkLoc) { Node node = null; synchronized (nodesAtMaxLevel) { if ((node = clusterMap.getNode(networkLoc+"/"+host)) == null) { node = new NodeBase(host, networkLoc); clusterMap.add(node); if (node.getLevel() < getNumTaskCacheLevels()) { LOG.fatal("Got a host whose level is: " + node.getLevel() + "." + " Should get at least a level of value: " + getNumTaskCacheLevels()); try { stopTracker(); } catch (IOException ie) { LOG.warn("Exception encountered during shutdown: " + StringUtils.stringifyException(ie)); System.exit(-1); } } hostnameToNodeMap.put(host, node); // Make an entry for the node at the max level in the cache nodesAtMaxLevel.add(getParentNode(node, getNumTaskCacheLevels() - 1)); } } return node; }
以上创建节点任务映射缓存的目的就是为调度任务提供本地性选择策略。最后选择的 Task 只是一次尝试运行。需要注意的是,JobTracker 中有一个 org.apache.hadoop.mapred.TaskInProgress
类和 org.apache.hadoop.mapred.TaskTracker.TaskInProgress
类不是同一个。一个Task Attempt在某个节点上运行失败之后, 调度器便不会再将同一个Task的Task Attempt分配给该节点; 而
一个Task Attempt被杀掉后, 仍可能被调度到同一个节点上运行。
TaskTracker 中的形成的一个 TIP 实体,其实只是 JobTracker 中TaskInProgress的一个 TaskAttempt 任务, 是TaskInProgress 的一次任务尝试, 该尝试任务 Task(MapTask/ReduceTask) 会装入TaskAction 一起序列化通过RPC 从 JobTracker 流入 TaskTracker, TaskTracker 利用自己的org.apache.hadoop.mapred.TaskTracker.TaskInProgress
对 Task 进行重新封装,该内部TIP包含 Task, TaskRunner, TaskLauncher, TaskStatus 是Task真实运行状态和行为控制的数据结构,JobTracker 中的 org.apache.hadoop.mapred.TaskInProgress
只是含有一些运行过程的数据。
Task 分配
public synchronized Task obtainNewNodeLocalMapTask(TaskTrackerStatus tts, int clusterSize, int numUniqueHosts) throws IOException { if (!tasksInited) { LOG.info("Cannot create task split for " + profile.getJobID()); try { throw new IOException("state = " + status.getRunState()); } catch (IOException ioe) {ioe.printStackTrace();} return null; } int target = findNewMapTask(tts, clusterSize, numUniqueHosts, 1, status.mapProgress()); if (target == -1) { return null; } Task result = maps[target].getTaskToRun(tts.getTrackerName()); if (result != null) { addRunningTaskToTIP(maps[target], result.getTaskID(), tts, true); resetSchedulingOpportunities(); } return result; } // TaskInProgress public Task getTaskToRun(String taskTracker) throws IOException { if (0 == execStartTime){ // assume task starts running now execStartTime = jobtracker.getClock().getTime(); } // 创建一个任务尝试id,返回一个新的MapTask或者ReduceTask, 对于JobTracker 来说只是TIP中的一次尝试,对于TaskTracker来说就是一个真实运行的任务 // Create the 'taskid'; do not count the 'killed' tasks against the job! TaskAttemptID taskid = null; if (nextTaskId < (MAX_TASK_EXECS + maxTaskAttempts + numKilledTasks)) { // Make sure that the attempts are unqiue across restarts int attemptId = job.getNumRestarts() * NUM_ATTEMPTS_PER_RESTART + nextTaskId; taskid = new TaskAttemptID( id, attemptId); ++nextTaskId; } else { LOG.warn("Exceeded limit of " + (MAX_TASK_EXECS + maxTaskAttempts) + " (plus " + numKilledTasks + " killed)" + " attempts for the tip '" + getTIPId() + "'"); return null; } return addRunningTask(taskid, taskTracker); } // obtainNewNodeOrRackLocalMapTask // int target = findNewMapTask(tts, clusterSize, numUniqueHosts, maxLevel, status.mapProgress()); // obtainNewNonLocalMapTask // int target = findNewMapTask(tts, clusterSize, numUniqueHosts, NON_LOCAL_CACHE_LEVEL, status.mapProgress());
当需要从作业中选择一个MapTask时, 调度器会直接调用JobInProgress
中的obtainNewMapTask
等方法。 这些方法最终调用的是 findNewMapTask
该方法封装了所有调度器公用的任务选择策略实现。 其主要思想是优先选择运行失败的任务, 以让其快速获取重新运行的机会, 其次是按照数据本地性策略选择尚未运行的任务, 最后是查找正在运行的任务, 尝试为“拖后腿”任务启动备份任务。
具体步骤如下:
1. 合法性检查。 如果一个作业在某个节点上失败任务数目超过一定阈值或者该节点剩余磁盘容量不足, 则不再将该作业的任何任务分配给该节点。
// Check to ensure this TaskTracker has enough resources to // run tasks from this job long outSize = resourceEstimator.getEstimatedMapOutputSize(); long availSpace = tts.getResourceStatus().getAvailableSpace(); if(availSpace < outSize) { LOG.warn("No room for map task. Node " + tts.getHost() + " has " + availSpace + " bytes free; but we expect map to take " + outSize); return -1; //see if a different TIP might work better. }
这里采用的预估算法是一种简单的线性算法,通过已经运行完成的任务产生的数据量估算出其他同作业任务需要的磁盘空间
$$
estimatedTotalMapOutput = inputSize \times \frac{completedMapsOutputSize}{completedMapsInputSize} \times 2
$$
$$
estimatedMapOutputSize = \frac{estimatedTotalMapOutput}{numMapTasks}
estimatedReduceInput = \frac{estimatedTotalMapOutput}{numReduceTasks}
$$
其中, inputSize表示输入数据总量, completedMapsInputSize和completedMapsOutputSize 分别表示所有已经运行完成的Map Task的总输入数据量和总输出数据量, 最后乘2表示输出数据量会翻倍(保守估计)。如果估算到某个Map/Reduce Task产生的数据量或者输入的数据量超过某个TaskTracker剩余磁盘空间, 则不会将该Task分配给它。
nonRunning
- 从failedMaps列表中选择任务。 failedMaps保存了按照Task Attempt失败次数排序的TIP集合。 失败次数越多的任务, 被调度的机会越大。 需要注意的是, 为了让失败的任务快速得到重新运行的机会, 在进行任务选择时不再考虑数据本地性。
- 从nonRunningMapCache列表中选择任务。 采用的任务选择方法完全遵循数据本地性策略, 即任务选择优先级从高到低依次为node-local, rack-local和off-switch类型的任务。
- 从nonLocalMaps列表中选择任务。 由于nonLocalMaps中的任务没有输入数据, 因此无须考虑数据本地性。
runing
- 从runningMapCache列表中选择任务。 遍历runningMapCache列表, 查找是否存在正运行且“拖后腿”的任务, 如果有, 则为其启动一个备份任务。
- 从nonLocalRunningMaps列表中选择任务。 同步骤5, 从nonLocalRunningMaps列表中查找“拖后腿”任务, 并为其启动备份任务。
private synchronized int findNewMapTask(final TaskTrackerStatus tts, final int clusterSize, final int numUniqueHosts, final int maxCacheLevel, final double avgProgress) { if (numMapTasks == 0) { if(LOG.isDebugEnabled()) { LOG.debug("No maps to schedule for " + profile.getJobID()); } return -1; } String taskTracker = tts.getTrackerName(); TaskInProgress tip = null; // // Update the last-known clusterSize // this.clusterSize = clusterSize; if (!shouldRunOnTaskTracker(taskTracker)) { return -1; } // Check to ensure this TaskTracker has enough resources to // run tasks from this job long outSize = resourceEstimator.getEstimatedMapOutputSize(); long availSpace = tts.getResourceStatus().getAvailableSpace(); if(availSpace < outSize) { LOG.warn("No room for map task. Node " + tts.getHost() + " has " + availSpace + " bytes free; but we expect map to take " + outSize); return -1; //see if a different TIP might work better. } // When scheduling a map task: // 0) Schedule a failed task without considering locality // 1) Schedule non-running tasks // 2) Schedule speculative tasks // 3) Schedule tasks with no location information // First a look up is done on the non-running cache and on a miss, a look // up is done on the running cache. The order for lookup within the cache: // 1. from local node to root [bottom up] // 2. breadth wise for all the parent nodes at max level // We fall to linear scan of the list ((3) above) if we have misses in the // above caches // 0) Schedule the task with the most failures, unless failure was on this // machine tip = findTaskFromList(failedMaps, tts, numUniqueHosts, false); if (tip != null) { // Add to the running list scheduleMap(tip); LOG.info("Choosing a failed task " + tip.getTIPId()); return tip.getIdWithinJob(); } Node node = jobtracker.getNode(tts.getHost()); // // 1) Non-running TIP : // // 1. check from local node to the root [bottom up cache lookup] // i.e if the cache is available and the host has been resolved // (node!=null) if (node != null) { Node key = node; int level = 0; // maxCacheLevel might be greater than this.maxLevel if findNewMapTask is // called to schedule any task (local, rack-local, off-switch or // speculative) tasks or it might be NON_LOCAL_CACHE_LEVEL (i.e. -1) if // findNewMapTask is (i.e. -1) if findNewMapTask is to only schedule // off-switch/speculative tasks int maxLevelToSchedule = Math.min(maxCacheLevel, maxLevel); // 先从第一层次host local即当前tracker本身可运行的数据局部性Map任务,让后就找第二层rack local即在同一个rack可以运行的任务 for (level = 0;level < maxLevelToSchedule; ++level) { List <TaskInProgress> cacheForLevel = nonRunningMapCache.get(key); if (cacheForLevel != null) { tip = findTaskFromList(cacheForLevel, tts, numUniqueHosts,level == 0); if (tip != null) { // Add to running cache scheduleMap(tip); // remove the cache if its empty if (cacheForLevel.size() == 0) { nonRunningMapCache.remove(key); } return tip.getIdWithinJob(); } } key = key.getParent(); } //如果只调度 node-local / rack-local, 则返回 // Check if we need to only schedule a local task (node-local/rack-local) if (level == maxCacheLevel) { return -1; } } // 调度 off-switch , 扫描所有顶层的节点 //2. Search breadth-wise across parents at max level for non-running // TIP if // - cache exists and there is a cache miss // - node information for the tracker is missing (tracker's topology // info not obtained yet) // collection of node at max level in the cache structure Collection<Node> nodesAtMaxLevel = jobtracker.getNodesAtMaxLevel(); // get the node parent at max level Node nodeParentAtMaxLevel = (node == null) ? null : JobTracker.getParentNode(node, maxLevel - 1); for (Node parent : nodesAtMaxLevel) { // skip the parent that has already been scanned if (parent == nodeParentAtMaxLevel) { continue; } List<TaskInProgress> cache = nonRunningMapCache.get(parent); if (cache != null) { tip = findTaskFromList(cache, tts, numUniqueHosts, false); if (tip != null) { // Add to the running cache scheduleMap(tip); // remove the cache if empty if (cache.size() == 0) { nonRunningMapCache.remove(parent); } LOG.info("Choosing a non-local task " + tip.getTIPId()); return tip.getIdWithinJob(); } } } // 3. Search non-local tips for a new task tip = findTaskFromList(nonLocalMaps, tts, numUniqueHosts, false); if (tip != null) { // Add to the running list scheduleMap(tip); LOG.info("Choosing a non-local task " + tip.getTIPId()); return tip.getIdWithinJob(); } // // 2) Running TIP : 运行中的task // if (hasSpeculativeMaps) { long currentTime = jobtracker.getClock().getTime(); // 1. Check bottom up for speculative tasks from the running cache if (node != null) { Node key = node; for (int level = 0; level < maxLevel; ++level) { Set<TaskInProgress> cacheForLevel = runningMapCache.get(key); if (cacheForLevel != null) { tip = findSpeculativeTask(cacheForLevel, tts, avgProgress, currentTime, level == 0); if (tip != null) { if (cacheForLevel.size() == 0) { runningMapCache.remove(key); } return tip.getIdWithinJob(); } } key = key.getParent(); } } // 2. Check breadth-wise for speculative tasks for (Node parent : nodesAtMaxLevel) { // ignore the parent which is already scanned if (parent == nodeParentAtMaxLevel) { continue; } Set<TaskInProgress> cache = runningMapCache.get(parent); if (cache != null) { tip = findSpeculativeTask(cache, tts, avgProgress, currentTime, false); if (tip != null) { // remove empty cache entries if (cache.size() == 0) { runningMapCache.remove(parent); } LOG.info("Choosing a non-local task " + tip.getTIPId() + " for speculation"); return tip.getIdWithinJob(); } } } // 3. Check non-local tips for speculation tip = findSpeculativeTask(nonLocalRunningMaps, tts, avgProgress, currentTime, false); if (tip != null) { LOG.info("Choosing a non-local task " + tip.getTIPId() + " for speculation"); return tip.getIdWithinJob(); } } return -1; }
/** * Adds a map tip to the list of running maps. * @param tip the tip that needs to be scheduled as running */ protected synchronized void scheduleMap(TaskInProgress tip) { if (runningMapCache == null) { LOG.warn("Running cache for maps is missing!! " + "Job details are missing."); return; } String[] splitLocations = tip.getSplitLocations(); // Add the TIP to the list of non-local running TIPs if (splitLocations == null || splitLocations.length == 0) { nonLocalRunningMaps.add(tip); return; } // 将任务添加到正在运行任务拓扑缓存中 for(String host: splitLocations) { Node node = jobtracker.getNode(host); for (int j = 0; j < maxLevel; ++j) { Set<TaskInProgress> hostMaps = runningMapCache.get(node); if (hostMaps == null) { // create a cache if needed hostMaps = new LinkedHashSet<TaskInProgress>(); runningMapCache.put(node, hostMaps); } hostMaps.add(tip); node = node.getParent(); } } } // 从tips集合中找到合适的任务 private synchronized TaskInProgress findTaskFromList( Collection<TaskInProgress> tips, TaskTrackerStatus ttStatus, int numUniqueHosts, boolean removeFailedTip) { Iterator<TaskInProgress> iter = tips.iterator(); while (iter.hasNext()) { TaskInProgress tip = iter.next(); // Select a tip if // 1. runnable : still needs to be run and is not completed // 2. ~running : no other node is running it // 3. earlier attempt failed : has not failed on this host // and has failed on all the other hosts // A TIP is removed from the list if // (1) this tip is scheduled // (2) if the passed list is a level 0 (host) cache // (3) when the TIP is non-schedulable (running, killed, complete) if (tip.isRunnable() && !tip.isRunning()) { // check if the tip has failed on this host if (!tip.hasFailedOnMachine(ttStatus.getHost()) || tip.getNumberOfFailedMachines() >= numUniqueHosts) { // check if the tip has failed on all the nodes iter.remove(); return tip; } else if (removeFailedTip) { // the case where we want to remove a failed tip from the host cache // point#3 in the TIP removal logic above iter.remove(); } } else { // see point#3 in the comment above for TIP removal logic iter.remove(); } } return null; }
reduce调度, reduce 调度的时机是在 Job 的MapTask 完成一定数目的时候才开始的,这个数目由参数
mapred.reduce.slowstart.completed.maps
控制,默认情况下, 当Map Task完成数目达到总数的5%.
completedMapsForReduceSlowstart = (int)Math.ceil( (conf.getFloat("mapred.reduce.slowstart.completed.maps", DEFAULT_COMPLETED_MAPS_PERCENT_FOR_REDUCE_SLOWSTART) * numMapTasks)); public synchronized Task obtainNewReduceTask(TaskTrackerStatus tts, int clusterSize, int numUniqueHosts ) throws IOException { if (status.getRunState() != JobStatus.RUNNING) { LOG.info("Cannot create task split for " + profile.getJobID()); return null; } /** check to see if we have any misbehaving reducers. If the expected output * for reducers is huge then we just fail the job and error out. The estimated * size is divided by 2 since the resource estimator returns the amount of disk * space the that the reduce will use (which is 2 times the input, space for merge + reduce * input). **/ long estimatedReduceInputSize = resourceEstimator.getEstimatedReduceInputSize()/2; if (((estimatedReduceInputSize) > reduce_input_limit) && (reduce_input_limit > 0L)) { // make sure jobtracker lock is held LOG.info("Exceeded limit for reduce input size: Estimated:" + estimatedReduceInputSize + " Limit: " + reduce_input_limit + " Failing Job " + jobId); status.setFailureInfo("Job exceeded Reduce Input limit " + " Limit: " + reduce_input_limit + " Estimated: " + estimatedReduceInputSize); jobtracker.failJob(this); return null; } // Ensure we have sufficient map outputs ready to shuffle before // scheduling reduces if (!scheduleReduces()) { return null; } int target = findNewReduceTask(tts, clusterSize, numUniqueHosts, status.reduceProgress()); if (target == -1) { return null; } Task result = reduces[target].getTaskToRun(tts.getTrackerName()); if (result != null) { addRunningTaskToTIP(reduces[target], result.getTaskID(), tts, true); } return result; } public synchronized boolean scheduleReduces() { return finishedMapTasks >= completedMapsForReduceSlowstart; }
调度数据更新的并发
调度器对整个集群资源数据的掌握情况是 TaskTracker
不断的通过心跳发来的,对集群资源数据的更改需要枷锁和同步,另外,每次调用 assignTasks
也是需要枷锁的,保证并发的 TaskTracker
任务分配最终是串行的。但是由于这是一个分布式系统,某个 TaskTracker
资源数据的变化无法立即反映到 JobTracker
和 TaskScheduler
, 因为这些数据其实是靠心跳来传输的,可能存在不一致。但是这种数据不一致性并不会导致致命错误,他和银行转账不一样。是可容忍的。
不像是基于数据库的应用,当前 TaskTracker
分配到任务以后,就可以立即减少数据库中它的资源数量,另一个 TaskTracker
在调用 assignTasks
的时候就可以立即获得真实的集群资源使用情况,而不是有延迟的或者虚假的,保证数据更新的一致性。